As OpenAI’s GPT-4 reportedly cost over $100 million to train and Google’s Gemini Ultra likely came with an even heftier price tag, one thing has become crystal clear: artificial intelligence is getting smarter and exponentially more expensive. Today’s cutting-edge AI models consume resources at a scale that would have been unimaginable just five years ago, from specialized chips in short supply to energy consumption that rivals that of small nations.
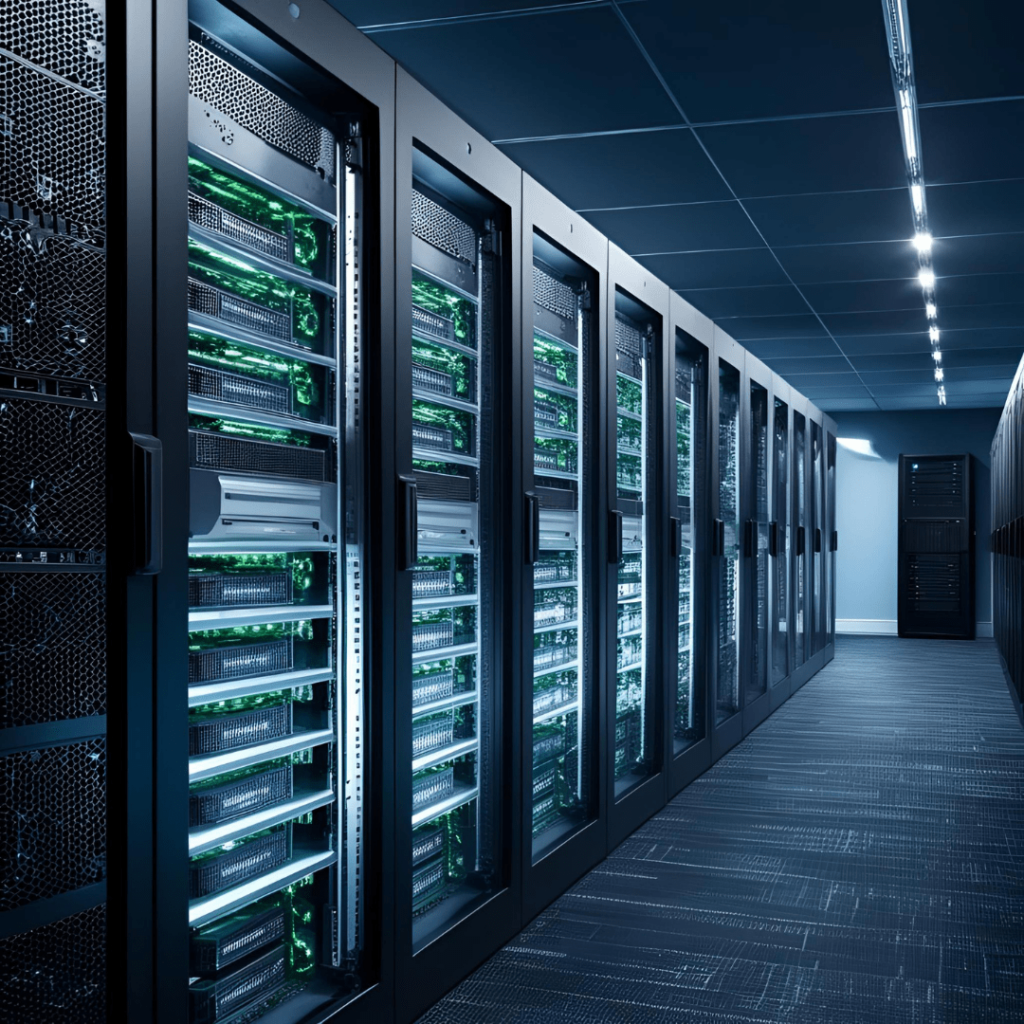
Behind the flashy demos and impressive capabilities lies an uncomfortable truth for the tech industry: intelligence has a rising price tag. As we stand at this critical inflection point, both established tech giants and ambitious startups face the same fundamental question: How sustainable is this trajectory of bigger, better, and significantly more costly AI?
In this post, we’ll explore why AI development costs continue to skyrocket, what this means for innovation and competition, and whether there’s a ceiling to how much we’re willing to invest in artificial minds. Whether you’re an investor trying to make sense of valuations, a developer wondering about the future of the field, or simply curious about where this technology is headed, understanding the economics behind AI advancement is essential to grasping what comes next.
The Current Cost Landscape
When we talk about AI becoming more expensive, what exactly are we paying for? Let’s break down where all that money goes.
First, there’s the computing infrastructure. Modern AI systems don’t run on ordinary computers – they need specialized hardware called GPUs (Graphics Processing Units) or TPUs (Tensor Processing Units). These aren’t your everyday computer parts. A single advanced AI chip can cost thousands of dollars, and the largest AI companies use thousands of these chips connected together. NVIDIA, the company making most of these chips, has seen its value soar precisely because these components are in such high demand.
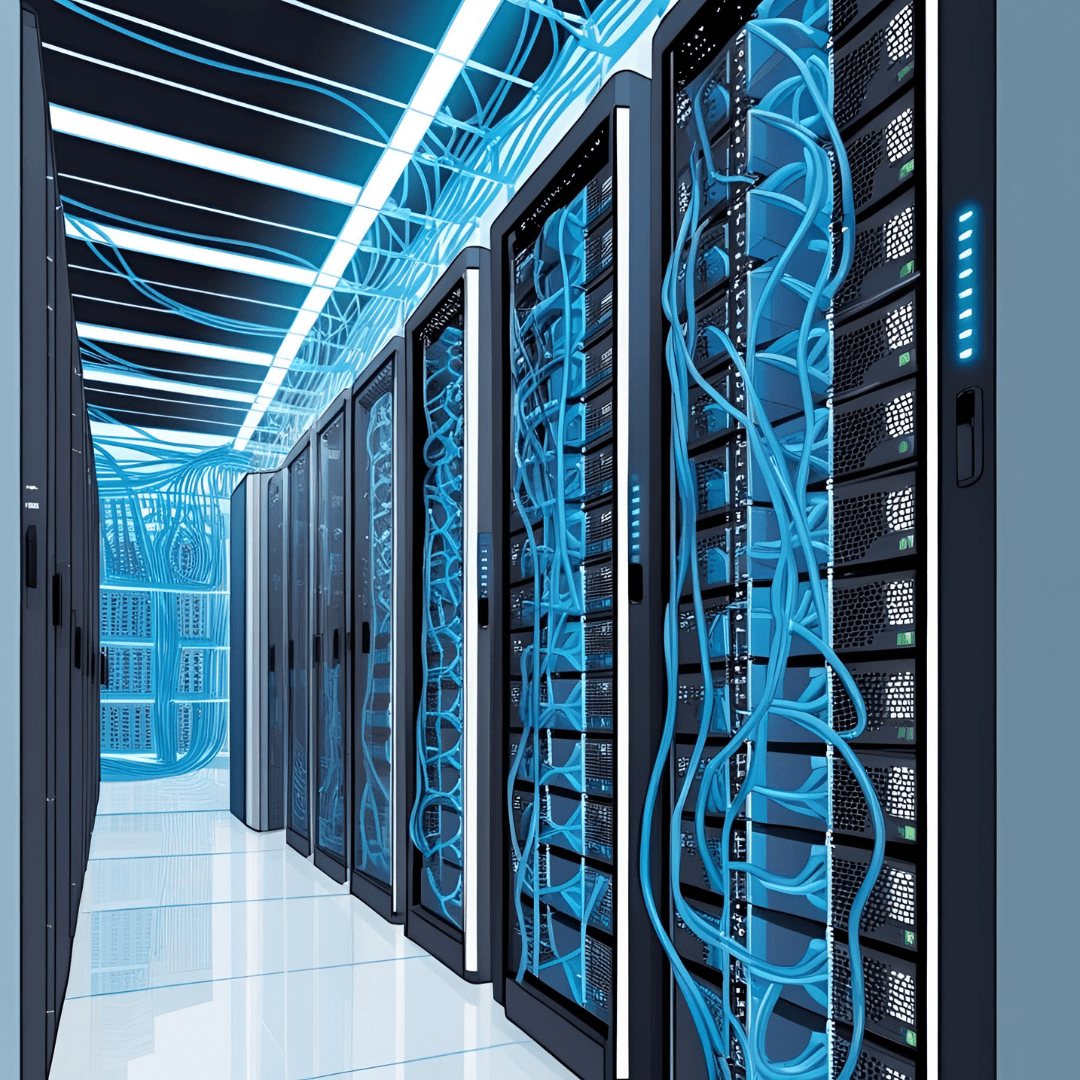
Next comes the energy bill. Training a large AI model uses enough electricity to power hundreds of homes for a year. For example, training GPT-3 (an earlier version of ChatGPT) reportedly consumed enough energy to power about 120 U.S. homes for a year. The newest models use even more power.
Then there’s the people factor. AI researchers with the right skills can command salaries of $300,000 to $500,000 per year or even more. Top AI scientists might earn millions annually. Companies are constantly competing to hire these experts, driving salaries ever higher.
Let’s look at some real numbers. Microsoft invested $10 billion in OpenAI. Google’s parent company Alphabet spent over $31 billion on research and development in 2023, with a significant portion going to AI. Meta (formerly Facebook) announced it would spend around $35 billion on AI infrastructure in 2024 alone.
These aren’t just big numbers – they represent a fundamental shift in what it takes to compete in the AI space.
Why Costs Are Escalating
The rising cost of AI isn’t just inflation – there are specific reasons why building these systems gets more expensive every year.
The main driver is size and complexity. AI models keep getting bigger. GPT-3 had 175 billion parameters (the adjustable parts that help the AI learn), while GPT-4 reportedly has over a trillion. Each time we make these models bigger, the computing resources needed grow dramatically – not just doubling but increasing by many times.
It’s like building skyscrapers – a 100-story building doesn’t just cost twice as much as a 50-story building. The taller you go, the more complex the engineering challenges become, and costs rise accordingly
Competition is another big factor. Major tech companies are racing to build the most powerful AI, and this race has created shortages of critical components. When NVIDIA released its advanced H100 AI chips, they sold out almost immediately, with some buyers willing to pay far above the retail price.
Energy requirements are also increasing. Training more complex models needs more electricity, and running these models (called “inference”) uses energy too. As more people use AI tools like ChatGPT, the energy needed to keep them running grows substantially.
Finally, there’s the talent war. With only so many people who understand how to build these systems, companies offer increasingly generous compensation packages to attract and keep top talent. When an AI researcher with the right expertise can easily earn seven figures, the human cost of development becomes significant.
The Economic Implications
What does this expensive trend mean for the AI industry and our economy?
For starters, it’s creating a major barrier to entry. When it costs hundreds of millions or even billions of dollars to build competitive AI systems, only the largest companies can afford to play. This leads to market consolidation – fewer companies controlling more of the technology.
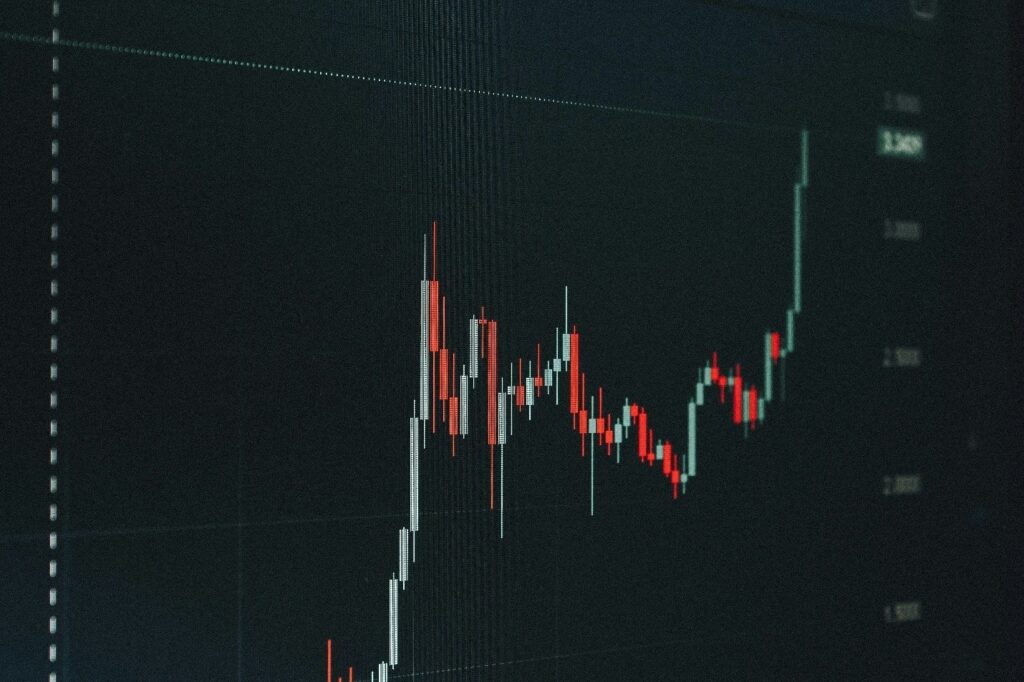
We’re already seeing this happen. Five years ago, dozens of startups were developing their own large language models. Today, most have either been acquired by larger companies or have shifted to building applications on top of models created by tech giants.
These high costs also impact which problems get solved. Companies need to prioritize applications that can generate enough revenue to justify the enormous investment. This might mean focusing on business tools or entertainment rather than addressing important but less profitable challenges like climate science or rare disease research.
There are environmental considerations too. Data centers already account for about 1-2% of global electricity use, and AI is driving that percentage higher. As energy consumption increases, so does the carbon footprint of AI development – unless companies make deliberate choices to use renewable energy sources.
For consumers and businesses, these costs eventually get passed down. While many AI tools start with free tiers, companies need to recoup their massive investments somehow. We’re already seeing subscription models for advanced AI features, and prices are likely to increase as development costs rise.
Strategies for Sustainable AI Development
With costs climbing so rapidly, the industry is looking for ways to make AI development more sustainable. Here are some of the most promising approaches:
Making algorithms more efficient is a top priority. Instead of just building bigger models, researchers are finding ways to get more intelligence from smaller ones. This is similar to how phones became more powerful even as they got smaller – smart design beats brute force.
Specialized hardware solutions are also emerging. Traditional computing was designed for different kinds of tasks, but AI-specific chips can be much more efficient. Companies like NVIDIA, AMD, and even newcomers are developing chips specifically optimized for AI workloads.
Collaborative research models offer another path forward. Organizations like Hugging Face and EleutherAI have shown that open-source collaboration can produce impressive results without the resources of tech giants. By sharing research, data, and even pre-trained models, these communities distribute the cost while accelerating progress.
Some companies are developing smaller, specialized models rather than trying to build all-purpose systems. These “expert” models focus on specific domains like medicine or legal text, requiring less training data and computing power while sometimes outperforming general models in their specialty areas.
The Future Cost Trajectory
So what comes next? Will AI just keep getting more expensive forever?
Most experts predict that costs will continue rising in the short term. The race to build more capable AI shows no signs of slowing down, and the resources needed to train and run these systems will grow accordingly.
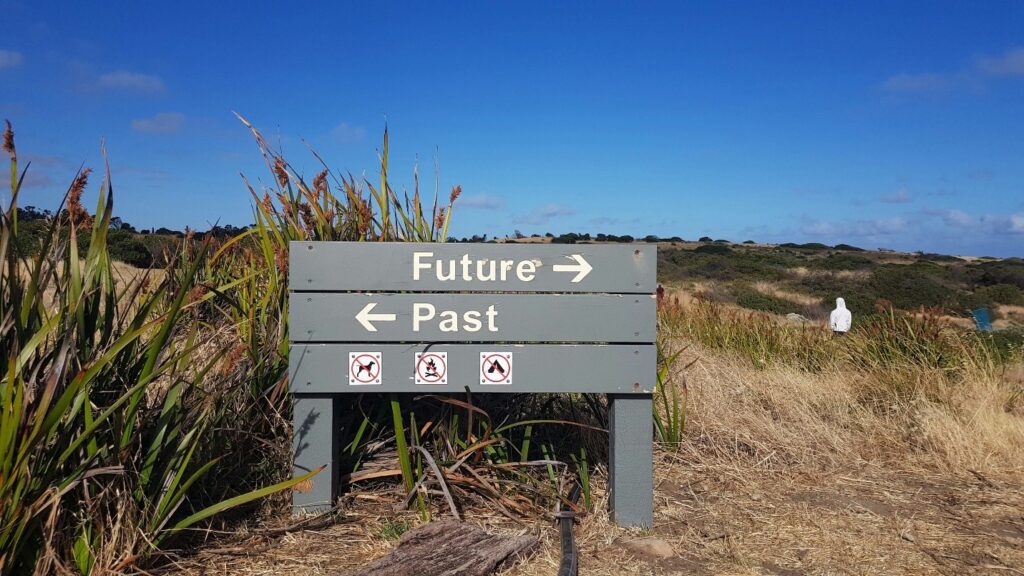
However, there are some potential game-changers on the horizon. Quantum computing, still in its early stages, could eventually provide exponential improvements in processing power. New algorithmic approaches might find shortcuts to intelligence that don’t require such massive models.
Some researchers are exploring “sparse models” that activate only relevant parts of the network for specific tasks, significantly reducing computational needs. Others are working on “once-for-all” training methods that can produce multiple specialized models from a single training run.
Economic models for AI development are evolving too. We’re seeing more hybrid approaches where companies share the basic research costs while competing on applications. Cloud computing providers are creating specialized AI infrastructure services, allowing smaller companies to rent rather than build expensive systems.
The market will likely find equilibrium. As costs rise, there’s more incentive to find efficiencies. Eventually, the law of diminishing returns will kick in – at some point, making a model twice as big won’t make it twice as useful.
Conclusion
The rising cost of artificial intelligence represents both a challenge and an opportunity. While the enormous resources required threaten to concentrate power in the hands of a few wealthy organizations, they also push the industry toward more creative, efficient solutions.
We’ve seen similar patterns in other technologies. Early computers filled entire rooms and cost millions in today’s dollars, but innovation eventually made them affordable and accessible. The difference with AI is the scale and speed of investment required, which dwarfs almost any previous technological revolution.
For AI to truly transform society in positive ways, we need to find paths that balance technical progress with economic and environmental sustainability. This might mean rethinking our approach to bigger models, encouraging more open collaboration, or developing entirely new computing paradigms.
The expense of intelligence is growing, but human ingenuity has a way of finding solutions to seemingly impossible challenges. The next chapter in AI’s story may not be about who can spend the most, but who can spend the smartest.